- AI Geekly
- Posts
- AI Geekly - Lies, Damned Lies and Benchmarks
AI Geekly - Lies, Damned Lies and Benchmarks
Entering the Age of AIQuarius...

Lies, Damned Lies, and Benchmarks
Welcome back to the AI Geekly, by Brodie Woods.

Gemini Must Gestate; G*?; Upsetting the AAPL cart; AMD’s David & Goliath III; Frowning Face; US Gov Underclocks NVDA
In brief: Google’s long-awaited GPT-4 killer, Gemini, remains long-awaited. Meanwhile, their AlphaCode 2 model looks promising, and reminds us a bit of OpenAI’s alleged Q* model, but compute costs must be contained. Apple’s new Open-Source AI framework will help developers better tap into the efficient parallel compute and memory sitting in modern Apple hardware. AMD’s new MI300 chips are finally out and along with Chinese sanctions on AI chips, might be one of the biggest threats to Nvidia’s dominance. Finally, we saw why the AI supply chain is critical as the sloppiness of human actors presents dangers.
Read on for the full story
AI News
Gemini Reaches for the Stars
Google announces long-awaited response to GPT-4

What it is: Google’s long-awaited Gemini multi-modal AI model was officially announced, albeit with limited access. Gemini comes in three flavors:
Gemini Nano —a smaller (1.8 or 3.3 Bn parameter) model designed for on-device/offline use on Google Pixel phones.
Gemini Pro —a more performant, online version that has replaced PALM 2 in powering Google’s Bard AI chatbot.
This is the version most people will engage with (sorry Canada, still no Bard)
Gemini Ultra —the real GPT-4 killer model we have been waiting for… still isn’t actually publicly available… Access to be expanded in purposely nebulous “early in 2024”.
What it means: We have been studying Gemini since “release”, its capabilities, and how it measures relative to expectations. While it is leaps and bounds ahead of its predecessor, PALM 2, what we’ve seen to date isn’t enough to supplant OpenAI’s nearly one-year-old GPT-4. Challenges include:
Generous ‘artistic license’ taken in the Gemini demo video (above), far more staged than at first glance and disingenuous, despite disclosures.
Questionable benchmarking comparing Gemini’s Ultra model to GPT-4, when reading the fine print, Google’s comparisons aren’t really apples-to-apples.
Limited public access to Gemini Ultra. Bard is Gemini Pro-powered (still can’t code), enterprise and developer access to Pro is Dec 13, but no timeline for Ultra, the model of interest.
Why it matters: Look. Gemini is fine. But Google’s Pichai is still slow walking this. Despite all the fanfare, Google delivered very little of what we expected for Gemini. They reannounced a product that we still don’t have access to, made some enhancements to Pixel devices (only!) and boosted an AI Chatbot that is honestly still not that great. Gemini Pro benchmarks haven’t been shared because they do not surpass OpenAI’s high watermark. The critical perspective is that Google has released another closed AI model, better than GPT 3.5, but with limited multimodal capabilities.
Catching OpenAI might be impossible: Google’s challenges reflect our concerns regarding delaying development, getting the AI innovation flywheel spinning. We are seeing shorter and shorter cycles between models, features, and modalities from OpenAI, and Open-Source players, whereas Google trails, as its own massive scale works against it. At this rate of acceleration, it may not be possible for Google to actually ever catch OpenAI. Perhaps the only option is to concede to Meta’s Open-Source approach.
Help me Open Source, You’re My Only Hope: With all of the money in the world, it seems Google can’t beat OpenAI. But if they embrace the OS community, they might just have a shot. As if to prove the point, France’s $2Bn Open-Source AI heavyweight Mistral dropped an 89 GB AI model shortly after Gemini’s release, with no comment or PR. This is what execution looks like in the AI Age.
Ok, But AlphaCode 2 Might be Impressive!
AlphaCode 2 shows us a Google Q*

What it is: Contemporaneous with the Gemini news, Google DeepMind announced its updated Gemini-powered AlphaCode 2 (AC2).
What it means: Given the foregoing on deceptive marketing practices —respect the hustle, but we’re moving to ‘trust, but verified’— we’re going to take Google’s data with a grain of salt. It appears that in certain conditions AlphaCode 2 may perform in the top 15% of coders. Notably, it features dynamic programming, breaking problems into subproblems repeatedly, but retaining overall scope.
Why it matters: Two things stand out here. In the span of a couple of short years, AlphaCode has gone from skills that are sub-human, to human, to superhuman coding AI. This pace creates interesting future possibilities. The second piece is the very Q*-like way in which Google describes how AC2 seems to reason about when and where to apply dynamic programming. This is promising, as we move to bridge LLMs’ underlying ‘glorified auto-complete’ underlying function (which is NOT a truth machine) to AI that can actually reason. ]
Upsetting the AAPL Cart
Apple releases machine learning framework optimized for its silicon

What it is: Outside of shoe-horning Apples’ Vision Pro into the Tech section of the Geekly, we’ve literally had zero reason to talk about them. We do greatly admire Cook’s shadow R&D silicon redesign that used iPhone releases for iterative chip improvements, eventually allowing it to converge mobile and laptop/desktop architecture (kicking Intel to the curb).
What it means: With the release of AAPL’s MLX Open-Source (yes!) framework, the company’s Machine Learning team making it easier for developers and enterprise to both train and run custom AI models on Apple’s aforementioned silicon. To be clear, this isn’t Apple’s response to Gemini, or ChatGPT or anything like that. They aren’t releasing their own AI model here, it’s a framework so we can build our own, with their gear.
Why it matters: Apple hardware is popular in North America (mobile and laptop) and in early testing has shown itself quite performant, particularly with its shared memory, enabling capabilities (like in-memory array storage) missing in comparable offerings (Pytorch, NumPy) upon which AAPL based its framework. In a world of chip shortages, and where inference represents an outsized share of AI workloads this move to untap a vast global supply of AI inference potential with large and low-latency memory (local) is an excellent way to increase demand for its hardware and provide another potential supply of AI hardware.
Tech News
AMD’s MI300 Chips Drops
Competition is heating up in the AI silicon saga

What it is: AMD’s MI300X (GPU only) and MI300A (GPU+CPU) are now available for its corporate clients. The MI300X reportedly perform similar to the H100 in model training, but 1.4x better on inference.
What it means: While model training horsepower is crucial, the massive amount of inference compute needed to run AI models (i.e. all the A100s, H100s, etc. running GPT-4, ChatGPT, etc.). over time eclipses the amount of compute used to train. As AI adoption increases, the demand for inference too will rise. Current bottlenecks to AI hardware from major players on the inference side largely come down to memory bandwidth and size (for now).
Why it matters: AMD is beginning to present a more formidable competitor to Nvidia. Those who’ve followed AMD over the decades know that being the underdog is in AMD’s blood (previous chip wars with Intel and Nvidia in particular). More companies are offering alternatives to Nvidia’s estimated 80% market share. Performant chips, feature parity to CUDA with ROCm, and an AI Software Platform for retail customers, the AMD riposte is looking very strong.
Supply Chains - Not Just For Inflating Egg Prices Anymore!
Poor user security habits threaten AI supply chain

What it is: Lasso Security researchers announced their findings of >1,500 API tokens after pouring through the results of a series of substring searches across several popular platforms, Hugging Face’s AI model and dataset hosting community. They were also able to access private repositories using deprecated API tokens.
What it means: This news reinforces the need for proper security and testing. The researchers were able to find the tokens hardcoded into users repositories (a practice that is generally discouraged unless working locally). Theoretically bad actors could use these API tokens to download and modify datasets and models for malicious purposes.
Why it matters: Hugging Face acts as the de facto community and repository for Open-Source AI with contributed datasets, models, and other assets from large public (Google, Meta, Microsoft, etc.) and private (ElutherAI, StabilityAI, Mistral AI, etc.). Therefore, it is critical for Hugging Face and the community to ensure that the supply chain is protected and clean. The good news here is that the Open-Source / Community ‘model’ worked. Ethical hackers identified the security hole and quietly informed the right people to patch this up.
Stop. Stop it. Just Stop it. STOP.
NVDA’s Efforts to circumvent China sanctions earn blunt warning from US Commerce Secretary
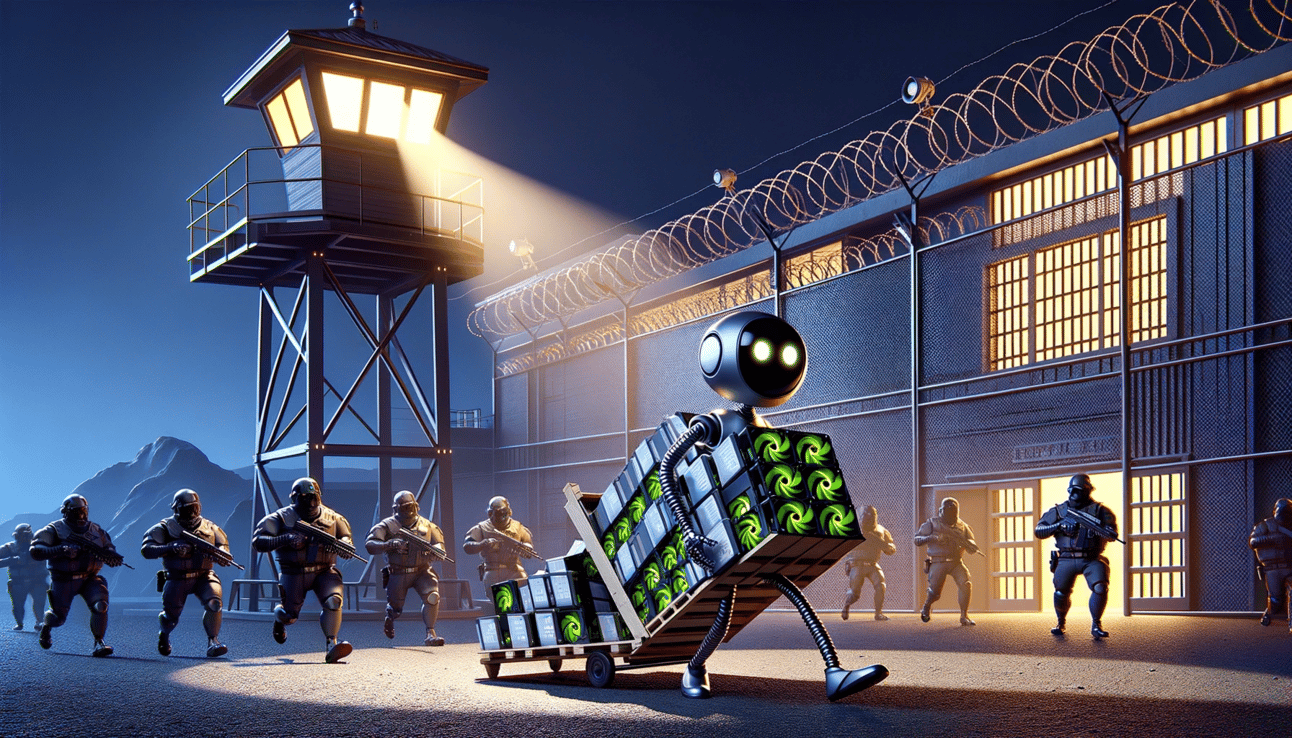
What it is: Over here at AI Geekly HQ, we’ve observed Nvidia’s efforts over the past several months to circumvent US Trade sanctions against China with a degree of curiosity. As new sanctions came out, NVDA would accommodate by tweaking its designs to limit specs as detailed, invariable coming-out with a new GPU for Chinese AI markets. Thus, we were little surprised when U.S. Commerce Secretary Gina Raimondo directly stated in an interview “If you redesign a chip around a particular cut line that enables [China] to do AI, I’m going to control it the very next day.”
What it means: Selling AI chips to China just got a lot harder. Nvidia had created custom, trimmed-down H800s and A800s when its H100s and A100s were prohibited. Then those were banned. Now they have received clear guidance to knock it off. CEO Jensen Huang noted that they are working closely with US regulators to find a compromise. We find it difficult to envision what such a compromise might look like.
Why it matters: We can’t blame them. Nvidia has 90% market share in China’s $7Bn AI Chip market and the region contributes ~20% of Nvidia’s revenue. China has also been very resourceful, so much so that the US has banned exports of Nvidia’s top-of-the-line RTX 4090 gaming graphics card best suited for AI workloads as reports indicated the cards were being purchased in bulk and reconfigured for AI use.
Before you go… We have one quick question for you:
How was the length of today's note? |
About the Author: Brodie Woods
With over 18 years of capital markets experience as a publishing equities analyst, an investment banker, a CTO, and an AI Strategist leading North American banks and boutiques, I bring a unique perspective to the AI Geekly. This viewpoint is informed by participation in two decades of capital market cycles from the front lines; publication of in-depth research for institutional audiences based on proprietary financial models; execution of hundreds of M&A and financing transactions; leadership roles in planning, implementing, and maintaining of the tech stack for a broker dealer; and, most recently, heading the AI strategy for the Capital Markets division of the eighth-largest commercial bank in North America.
Glossary
Gemini: A multi-modal AI model developed by Google, designed to process and integrate various types of information including text, images, audio, video, and code.
Gemini Nano: A smaller version of the Gemini AI model, optimized for on-device or offline use, particularly in Google Pixel phones.
Gemini Pro: An online version of the Gemini AI model, replacing PALM 2 in powering Google’s Bard AI chatbot, designed for more performant tasks.
Gemini Ultra: The most advanced version of Google's Gemini AI model, intended to perform highly complex tasks and considered a potential competitor to GPT-4.
PALM 2: An earlier AI model by Google, replaced by Gemini Pro in powering Google's Bard AI chatbot.
Bard: A chatbot by Google, powered by the Gemini Pro AI model, designed to assist with various tasks but without coding capabilities.
OpenAI: An AI research and deployment company, known for developing the GPT series of language models, including GPT-4.
GPT-4: A large multimodal language model developed by OpenAI, known for its advanced natural language understanding and generation capabilities.
Open Source (OS): A type of software where the source code is released under a license that permits users to study, change, and distribute the software to anyone for any purpose.
Mistral: A French AI company, notable for its contribution to the open-source AI community and its large AI models.
AlphaCode: An AI program developed by Google DeepMind that specializes in writing and understanding computer code.
Google DeepMind: A subsidiary of Alphabet Inc. and a research lab specializing in artificial intelligence, known for developing AlphaCode and part of the Gemini project.
AMD MI300 Chips: Advanced Micro Devices' (AMD) AI-focused chips, designed for both model training and inference tasks in AI applications.
CUDA: A programming model by Nvidia that allows developers to use GPUs for general purpose processing.
ROCm: AMD's open-source platform for GPU-based computing in high-performance and machine learning applications.
Nvidia (NVDA): A technology company known for its graphics processing units (GPUs) and AI computing hardware.
Hugging Face: A company offering a platform for sharing and collaborating on machine learning models, particularly focused on natural language processing.
API Token: A unique identifier used in programming to authenticate a user, developer, or calling program to an API (Application Programming Interface).
Sundar Pichai: CEO of Alphabet Inc. and its subsidiary Google, overseeing the development of products like Gemini.
Jensen Huang: CEO of Nvidia, known for leading the company's efforts in AI and graphics technologies.