- AI Geekly
- Posts
- AI Geekly: Alignment Check
AI Geekly: Alignment Check
Are we all on the same page?

Welcome back to the AI Geekly, by Brodie Woods, brought to you by usurper.ai. This week we bring you yet another week of fast-paced AI developments packaged neatly in a 5 minute(ish) read

In this week’s Geekly, we share some more views compiled as part of our 2025 Outlook series. In the prior note, we introduced our expectations for the year ahead including key themes and advances around Artificial General Intelligence (AGI). This week, we take inventory of the key players in the AI space. We examine their actions to date, their motives, and their willingness to collaborate, effectively an effort to measure the overall alignment of these players relative to broader societal goals. It’s similar to how AI companies themselves endeavor to ensure the alignment of their AI models with human ethics (or Superalignment), we’re simply distilling the process one order higher. Read on below!
Alignment Check - Who Can We Trust?
Quantifying collaboration and vibes
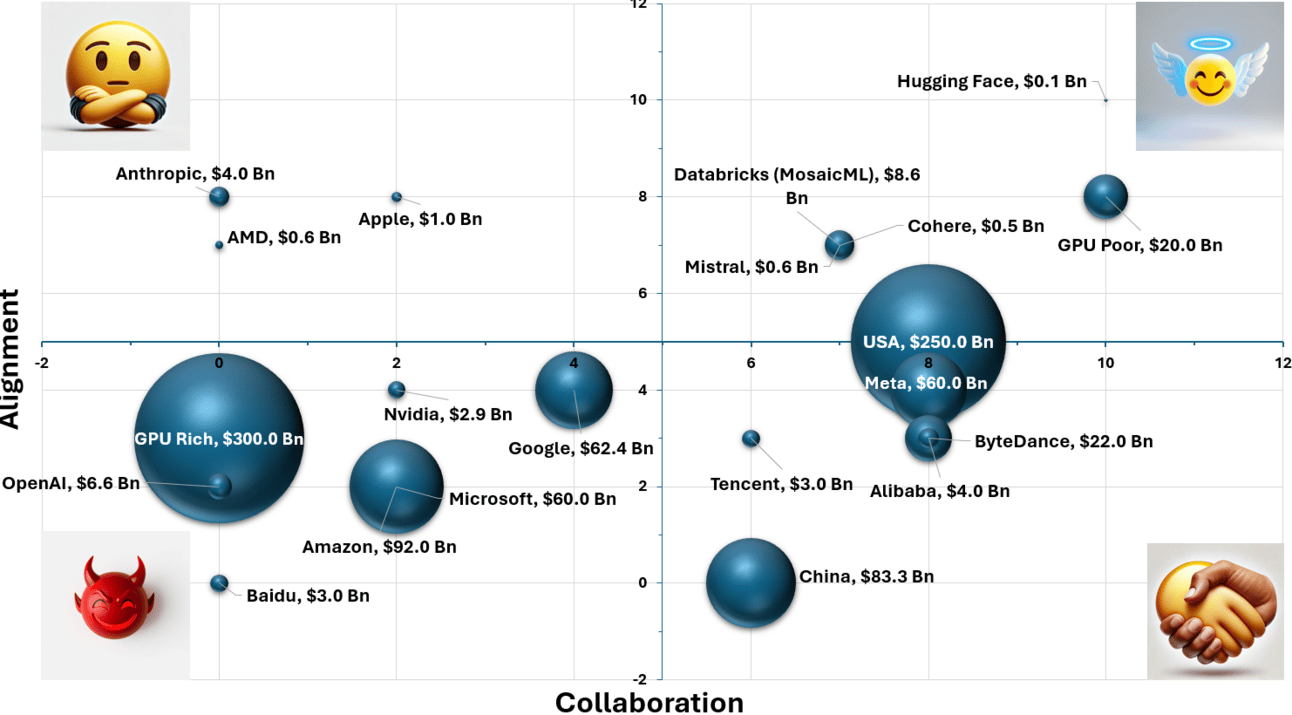
Source: internal estimates, company reports
In the chart above, we’ve done our best to quantify certain key features of the major players in the AI space on three specific metrics: open/closed source, alignment, and wallet. Parties embracing a more open, collaborative approach skew to the right along the X-axis while those preferring to play their cards closer to the vest fall on the left. Alignment, our measure of “vibe” and perhaps more broadly whether the entity has the common good and societal best interests at heart (rising up on the Y-axis) or whether it is more inclined to follow its own self-interest (down on the Y). Bubble size represents size of wallet (2025E AI capex is used, though there’s an argument for using available cash).
This ugly chart helps to set the stage for an examination of some 2025 themes and how we might think about them relative to the overall state of play. The AI race makes strange bedfellows. In particular, the peculiar alliance between the Open Source community, Local AI (i.e. on-device, on-PC, on-premises) + GPU Poor (the majority of people and companies who do not own millions of GPUs) and China and/or Meta. It’s a little weird, so we’ll explain:

Open Source: a loose community of practitioners, academics, companies, researchers, developers, and hobbyists who independently research and develop new AI techniques, tools, hardware, theories, models, etc. and share this information publicly —accelerating development and benefitting the public good. Not only does this community include groups like Stanford AI lab in the U.S., AMII and Vector in Canada, but can include companies as well, like Hugging Face (the de facto host of most open-source AI models), and researchers from Databricks, Cohere, Mistral, Nvidia, Microsoft and more! Yes, the closed shops sometimes contribute despite keeping their best models internal. They also shamelessly use the product of open-source research in their closed-source products —as we’ve previously mentioned, OpenAI and others productionized the concepts of Chain-of-Thought and Reflexion first posited by open-source researchers and baked this into the reasoning process in their o-series of reasoning models as part of their Test Time Compute evolution. Contrast this with Closed Source, the approach taken by the likes of OpenAI, Anthropic and the Big Tech players (ex-Meta) whereby advances and new techniques are closely guarded as trade secrets under a profit-driven motive.

Local AI: favored both by the open-source community (for flexibility and affordability) and regulated industries (Finance and Healthcare must often keep data on-premises) Local AI generally refers to the use of AI hardware at-or-near the use case. This includes things like Apple Intelligence on iOS 18 devices (where an on-device LLM handles certain tasks), hobbyist users with consumer cards like the Nvidia RTX 4090 running AI models on their PCs, and companies who host their own GPU servers for running fraud detection models (Nvidia’s Project Digits, discussed below is another example). Local AI allows practitioners to maintain control of costs and manage sensitive data without concern for prying eyes, though this comes at the cost of higher capital investment and limited scalability. Contrast this with Cloud AI and hosted AI solutions, where users only rent hardware (generally) and a third-party manages the AI and data infrastructure. While more convenient in certain cases (and cheaper on a pay-as-you-go rate) Cloud also has drawbacks —privacy, security, and third-party reliance to name a few.

GPU Poor: In a corporate context, this would refer to companies with limited access to GPUs. Be those small-businesses or larger enterprises, who don’t have access to thousands of expensive GPUs to train AI models or complete AI inference tasks. This also stretches out more broadly to include society in general, as most individuals do not possess clusters of $100,000 Nvidia GPUs. Contrast this with the GPU Rich, the OpenAIs, Microsofts, Googles, AWSs, etc. of the world, flush either with NVDAs desirable series of GPUs running proprietary CUDA software or homebrewed chips (like AWS’ Trainium and Google’s Trillium).

China: Ok, with all the foregoing laid out, how does China fit in? Well, more accurately, China’s version of Big Tech has largely embraced open source. ByteDance, Alibaba and Tencent are responsible for the creation of several of the most popular and performant open-source AI models: the DeepSeek, Qwen/QwQ, and Hunyuan families of models, respectively. These models are brawlers. They go toe to toe with the best closed-source models available today (maybe not OpenAI’s new o3 model yet, but it’s still unreleased). While this might seem impressive given the fact that the U.S. has been employing sanctions against China in efforts to slow its AI ambitions, reporting has shown these sanctions are easy to circumvent. Further, the models have clearly been trained using synthetic outputs from leading American models in violation of the TOS (when asked, these Chinese models will often claim to be Anthropic’s Claude or OpenAI’s GPT-4). Despite the questionable provenance of the training data, the open-source community is indebted to these companies. Their ingenuity in reverse-engineering the Test-Time-Compute employed by OpenAI’s o-reasoning models too is a boon. These are meaningful contributions, but a couple of things to note. 1) This is replication/imitation, not innovation. 2) There are meaningful security risks deploying models under the purview of the PRC, as we have discussed in previous notes. While we expect the same is true with regard to Llama models (i.e. that U.S. government back-doors have been built in), our readership would generally be at greater risk from PRC-exploitation of said vulnerabilities than from the U.S.
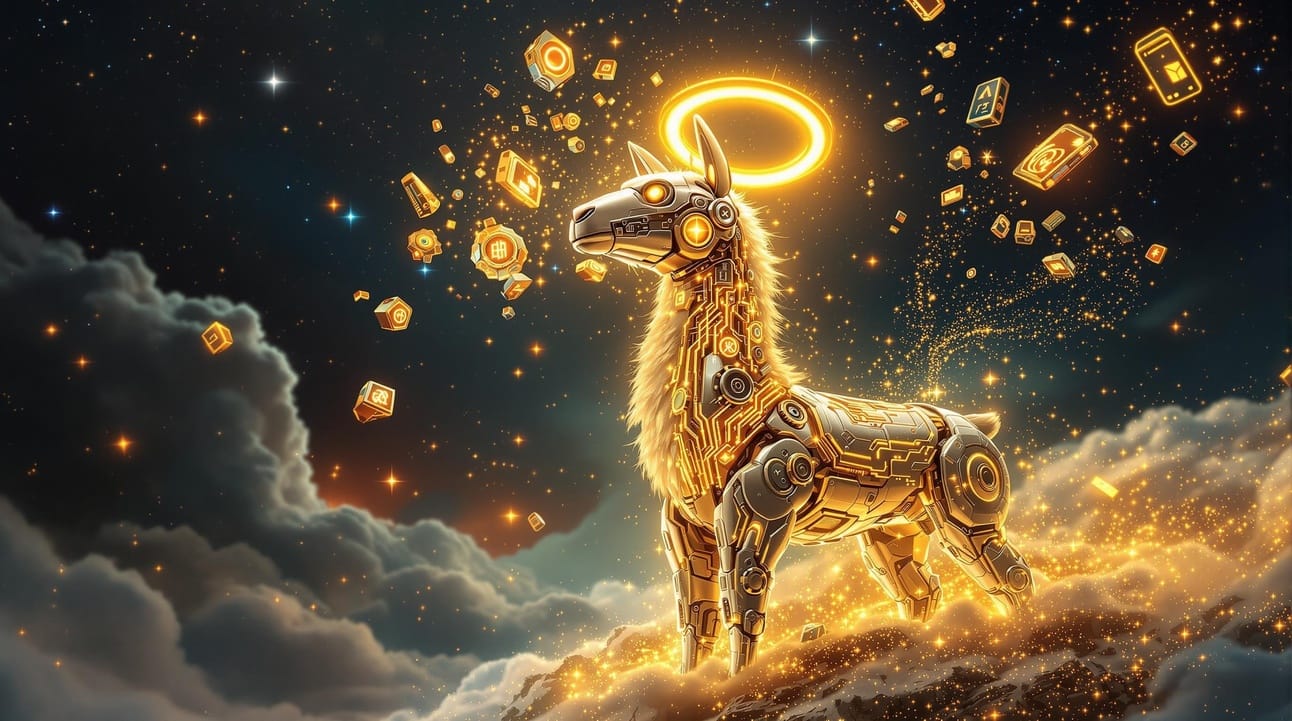
Meta: Like the Chinese tech co’s mentioned above, Meta has taken the open-source route, though perhaps for different reasons. Meta’s Llama series of models are some of the most popular open-source LLMs, so much so that one of the more popular local AI reddit communities, r/LocalLLaMA, was established around the initial release of Llama. Rather than stemming from some sort of benevolence or embrace of public good, Meta’s motivations for the release of Llama as an open-source model appear more in line with a scorched earth approach to the investment within the competitive environment. What do we mean? Every time Llama releases one of its Llama models, it’s within spitting distance of the current top model from competitors like OpenAI, Anthropic, etc. but releasing them for free, publicly diminishes the value of competitors’ models to nil —it’s free to download, so why pay for OpenAI’s model? Meta can afford to do this because it has billions of dollars in cash on its balance sheet. It can keep erasing the value of all of the research that OpenAI et al. do for as long as it wants, and in so doing cripple its competitors over time in a war of attrition. It just so happens that a happy side effect of this long-game corporate strategy is a public good.
CES Rockstar Performance
Nvidia lights-up the stage at CES 2025

What it is: We would be remiss if we didn’t mention the announcements from Nvidia at CES 2025. As expected, the company launched its $1,999 RTX 5090 flagship card with 32 GB of VRAM. As a little surprise, it also announced Project Digits, a $3,000 128 GB RAM mini-AI PC for small-scale AI use by developers, researchers and users. The company also released its COSMOS world model as part of a major push to usher in a new era of modern AI-enabled robotics (recall we expect AI robots to become a bigger theme in 2025).
What it means: The consumer-focused RTX 5090 comes with only 32 GB of VRAM in order to prevent cannibalization of the company’s professional and datacenter card segments, which are built on the same silicon, use many of the same components, but come with much higher VRAM (usually 60-80 GB). Project Digits was the big surprise and while it seems like Nvidia is extending an olive branch to enthusiasts and researchers with limited budgets, the $3,000 unit has several drawbacks that make it less appealing than at first glance. While the large 128 GB RAM capacity is welcome, and can fit versions of many of today’s larger LLMs, the compute of the unit is very low (on par with an RTX 3070) and the memory bandwidth (a key bottleneck as mentioned last week) appears to be quite low as well.
Why it matters: So while at first glance CES’ announcements should have been a boon to the “GPU Poors” and Open-Source community (particularly Project Digits), when we dig into the numbers, it’s clear that the company continues to cater to the much more lucrative Closed-Source, Enterprise, and Hyperscaler professional markets. The 5090 is a good card, predictably neutered for AI. Project Digits is a fun toy for experimenting, and a great way for Nvidia to monetize some of its more poorly binned Blackwell silicon dies, but it is not the “Democratization of AI hardware” that some outlets have reported.
Ace up the sleeve: The hidden benefit to having to do more with less is that it teaches discipline, adaptability, and resourcefulness. As the Open Source and GPU Poor make do with less, new techniques are developed to optimize AI for weaker hardware, evolving its applicability to a wider set of use cases as its deployability extends to a wider set of edge devices.
Before you go… We have one quick question for you:
If this week's AI Geekly were a stock, would you: |
About the Author: Brodie Woods
As CEO of usurper.ai and with over 18 years of capital markets experience as a publishing equities analyst, an investment banker, a CTO, and an AI Strategist leading North American banks and boutiques, I bring a unique perspective to the AI Geekly. This viewpoint is informed by participation in two decades of capital market cycles from the front lines; publication of in-depth research for institutional audiences based on proprietary financial models; execution of hundreds of M&A and financing transactions; leadership roles in planning, implementing, and maintaining of the tech stack for a broker dealer; and, most recently, heading the AI strategy for the Capital Markets division of the eighth-largest commercial bank in North America.