- AI Geekly
- Posts
- AI Geekly: A Wave, A Horse, and A Cloud
AI Geekly: A Wave, A Horse, and A Cloud
Enterprise AI solutions firm up

Welcome back to the AI Geekly, by Brodie Woods, brought to you by usurper.ai. This week we bring you yet another week of fast-paced AI developments packaged neatly in a 5 minute(ish) read.

TL;DR Clippy Rides Wave 2; A “Qwenstion” of Trust; On LlamaCloud Nine
This week we have a host of AI news updates from the likes of Microsoft, Alibaba, and LlamaIndex. This week’s issue is a little more geared towards our enterprise readers as the updates this week are firmly focused on the world of “how do we use AI to actually make money?” To start, Microsoft announced the largest update to its Microsoft 365 Copilot offering yet, addressing several of the pain points that have slowed adoption of its tools in corporate environments —we’ll tell you if we think they’re on the right track. Next, we look at a pile of new models from Alibaba as part of its Qwen family of open-source models. While Alibaba is quick to play-up its love of open source, we question the company’s motives and how much control the Chinese government exercises over the company’s AI aspirations… There may be something nefarious inside. Finally, we look at LlamaIndex’s improvements to LlamaCloud that make its enterprise-focused data retrieval pipeline tool much more useful by processing data beyond simple text. Read on below!
Satya Doubles Down
Microsoft announces Copilot “Wave 2” with host of new features

What it is: Microsoft is expanding its AI-powered Copilot platform with a suite of new features and enhancements across its Microsoft 365 product line (formerly Office). Dubbed "Wave 2," this update introduces Copilot Pages – a collaborative AI-powered workspace, significant improvements to Copilot integration within existing Microsoft 365 apps (Excel, PowerPoint, Teams, Outlook, and Word), and the release of Copilot agents designed to automate business processes. The Copilot agents function very similar to the custom GPTs released as part of ChatGPT many months ago, but with permission-based access to company data collateral (a must for enterprise customers).
What it means: This Copilot refresh comes at a critical time. As investors continue to question the return on billions of dollars poured into Generative AI, by MSFT in particular, and as recent media coverage of M365 Copilot has highlighted many of its shortcomings —the Windows maker needs a win. This update may very well be that. From the demos we’ve seen, the enhanced functionality built into Excel, PowerPoint and Outlook seem to resolve several of the limitations driving user complaints. For instance, in PowerPoint users now have finer-grained control of slide generation, no longer bound to whatever nonsense the AI spins-up based on a singular prompt, the process is now a much-needed back-and-forth conversation between the human and Copilot and even generates decks in the company’s slide template format!
Why it matters: Microsoft's substantial investment in Copilot and its continuous development cycle, marked by this "Wave 2" release, demonstrate CEO Satya Nadella’s commitment to generating a meaningful return from his investment in OpenAI over the past couple of years. Success for Microsoft depends on convincing businesses to adopt these AI-powered tools not as mere add-ons, but as indispensable components of their operations. Demonstrating quantifiable value, in the form of improved productivity, streamlined workflows, and reduced costs, is essential to persuading businesses to embrace AI and solidify Microsoft's position as the go-to provider of enterprise AI solutions.
Reasoning like o1: One thing that stands out from the demos is the thought process and planning that Copilot now exposes to the user. It all looks eerily similar to the Chain of Thought reasoning and Reflection (both capitalized as these are specific methods of improving LLM outputs within the discipline) displayed to users when using OpenAI’s new reasoning models o1-preview and o1 mini, discussed in last week’s Geekly. This makes sense on a few fronts: the timing of the release of Wave 2 coincides with the release of o1, and the performance of Wave 2 Copilot seems to benefit considerably from thinking in steps and reflecting on its outputs just like with the o1 family of models. We’re glad to see this functionality making its way into the M365 suite immediately upon release of same by OpenAI.
Three Syllables
O-pen source? or Tro-jan horse?

What it is: Alibaba Cloud has released Qwen2.5, its latest suite of open-source large language models (LLMs), along with specialized models for coding (Qwen2.5-Coder) and mathematics (Qwen2.5-Math) in what may be the largest single drop of open-source AI models to date (over 40 models). This extensive release encompasses a wide range of model sizes, all trained on a massive dataset of up to 18 trillion tokens and boasting significant improvements in knowledge, coding capabilities, and mathematical reasoning, besting the current top dog in open source, Meta’s Llama 3.1, in benchmarks. The models support a context window (how much data the model can “hold in its head” at one time) of up to 128,000 tokens (~300 pages of text), can generate up to 8,000 tokens (6,000 words), and are multilingual, supporting over 29 languages (relatively high).
What it means: We applaud Alibaba's open-source approach. Offering these advanced models under the Apache 2.0 license is a significant contribution to the AI community and helps to challenge the dominance of closed-source models offered by companies like OpenAI and Google. The diverse model sizes, specialized capabilities, and comprehensive language support make Qwen2.5 a versatile and accessible toolset for a wide range of applications. The company's focus on community collaboration is in line with its espoused commitment to driving innovation through open-source AI development.
Why it matters: This democratization of AI tools has the potential to empower smaller companies and individual developers, allowing them to leverage advanced AI technology while avoiding many of the financial burdens and restrictions associated with closed-source models. The improved performance of Qwen2.5, particularly its advancements in instruction following, structured data understanding, and long-text generation, could lead to novel applications in code generation, and data analysis.
Just keep in mind: That said, we remain cautious about the use of AI models produced in China due to the potential for PRC influence. To ground this point further, we note this week’s US Court of Appeals hearing of the proposed TikTok ban wherein judges repeatedly made the distinction that Chinese government control is not simply foreign control, but “adversary control”. There are several ways that a bad actor like the PRC can utilize a corrupted model as an AI trojan horse (think of it like a supply chain attack). These include Data Poisoning where training data is contaminated with embedded biases —Tiananmen square? Democracy? Taiwanese independence? Or even more nefarious Backdoor Attacks where hidden triggers within the model can be activated to alter its behavior in malicious ways.
An example: think of a loan adjudication system with an LLM summarization layer: a compromised open-source LLM could be secretly corrupted during initial training with a backdoor to always approve loans with a special codeword address (e.g. 123 Baker Street). Exploiting this backdoor, bad actors could apply for and receive massive loans, that would normally be denied, allowing the malevolent party to drain financial institutions of large sums in carefully orchestrated exploit attacks.
More Than Just Text
LlamaIndex adds multimodal RAG
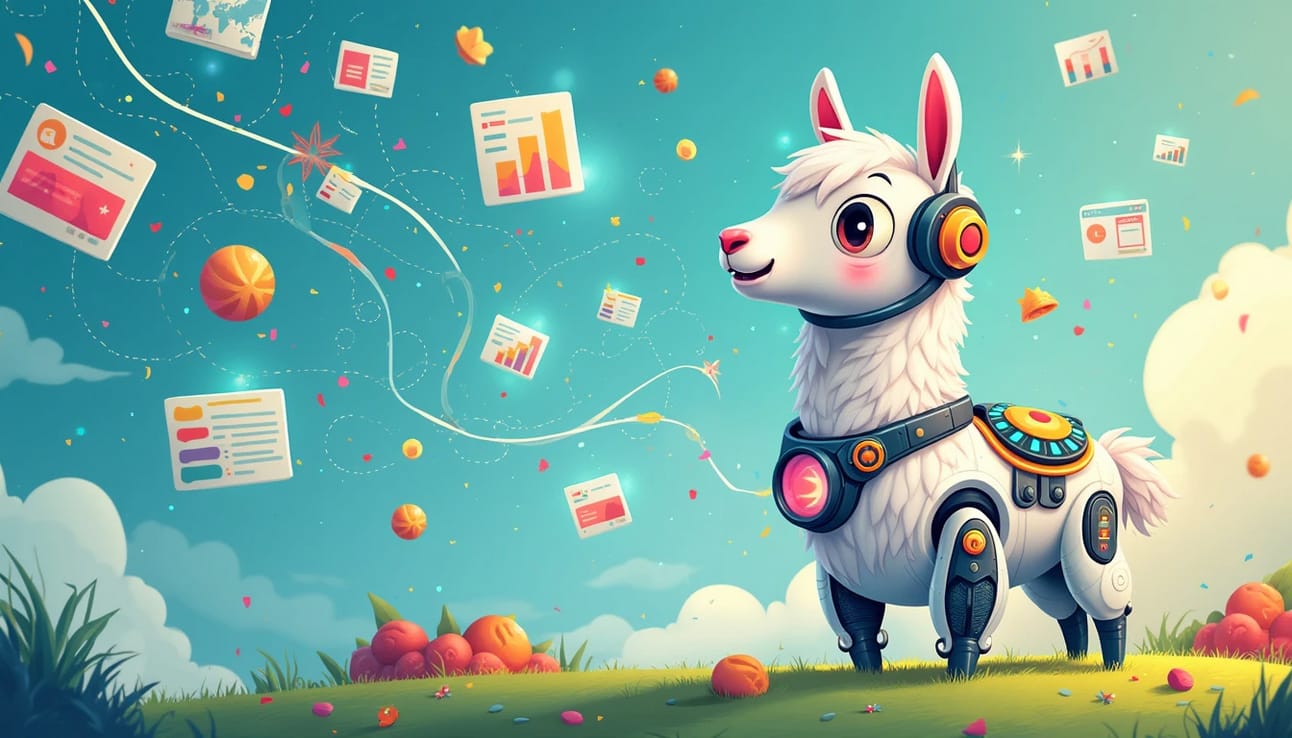
What it is: LlamaIndex, makers of LlamaCloud, an enterprise-focused Retrieval Augmented Generation (RAG) platform, has introduced a new multimodal capability (meaning the ability to absorb information beyond simple text). RAG systems are one of the most basic ways that enterprise users of LLMs are able to extract relevant responses from their expansive corpus of data assets. It makes sure that the AI responses provided to your queries have the context from your data. LlamaIndex’s multimodal enhancement allows developers to build RAG pipelines that seamlessly integrate text and image data from a variety of document types, including PDFs, presentations, and research reports. The solution addresses a significant limitation of traditional RAG systems that focus solely on textual information, leading to incomplete document understanding and increased inaccuracies in AI-generated responses.
What it means: LlamaCloud's multimodal feature simplifies the complex process of building and deploying multimodal RAG systems. By automating tasks like image extraction, indexing, and retrieval, LlamaCloud enables developers to focus on application logic and quickly create AI solutions capable of understanding and responding to both textual and visual information within documents. This feature reflects a practical reality of most companies’ data assets (i.e. they’re not just Word files), recognizing the importance of incorporating diverse data types to enhance AI systems' ability to comprehend and analyze real-world information.
Why it matters: LlamaIndex’s expanded offering significantly enhances the capabilities and accuracy of AI-powered knowledge assistants using their platform, particularly in enterprise settings where documents often contain a mix of text and visual elements. By providing a more comprehensive understanding of source materials, LlamaCloud's multimodal RAG pipelines can improve the quality of AI-generated insights, summaries, and reports.
Why do I care? We highlight this case for three reasons.
Extracting value from companies’ data assets is a lot more difficult than the problem seems on the surface due to the variety of structured and unstructured data and modalities of the data contained therein.
Even with tools like LlamaCloud there are serious limitations to what companies can accomplish to extract value from their data if they do not have rigorous data governance practices to ensure appropriate data quality.
A third aspect often overlooked is that real-time or recent data is not automagically incorporated into a RAG system, which means that often the most critical information for a workflow is inaccessible —this needs to be accounted for when designing a RAG system to ensure the utility of the tool.
Before you go… We have one quick question for you:
If this week's AI Geekly were a stock, would you: |
About the Author: Brodie Woods
As CEO of usurper.ai and with over 18 years of capital markets experience as a publishing equities analyst, an investment banker, a CTO, and an AI Strategist leading North American banks and boutiques, I bring a unique perspective to the AI Geekly. This viewpoint is informed by participation in two decades of capital market cycles from the front lines; publication of in-depth research for institutional audiences based on proprietary financial models; execution of hundreds of M&A and financing transactions; leadership roles in planning, implementing, and maintaining of the tech stack for a broker dealer; and, most recently, heading the AI strategy for the Capital Markets division of the eighth-largest commercial bank in North America.